-
Business Consulting
Our business consulting specialists offer a comprehensive blend of strategic advisory services. We assess the business, industry, operating model, synergy, skill sets and vision of the organisation and recommend the way forward
-
Digital Natives
Unlock growth with Grant Thornton Bharat's Digital Natives solutions. Customised support for tech-driven companies in healthcare, gaming, and more.
-
Digital Transformation Services
Grant Thornton’s digital transformation services help traditional businesses digitalise their business models with cloud technology, IoT consulting, app development and more DigiTech solutions.
-
Finance Transformation
Using a holistic approach, integrating digitalisation and digital transformation we help clients achieve transparency, control, governance, and faster decision making through real-time data within the business.
-
Human Capital Consulting
Our Human Capital Consulting team harnesses technology and industry expertise to assist in constructing adaptable organisations with transparency, fostering productive and value-driven workforces, and inspiring employees to engage meaningfully in their tasks.
-
Production Linked Incentive Scheme
Production-linked Incentive Scheme by the Indian government is aimed at boosting manufacturing. Grant Thornton Bharat offers varied services across sectors to help businesses avail of this scheme.
-
Public Sector Advisory
Our Public Sector Advisory team has focused streams, aligned with the core priorities of the Government of India. We are responsible for providing innovative and customized technical and managerial solutions.
-
Tech Advisory
We have amalgamated Digital Transformation, IT Advisory & Information Management and Analytics into a new offering, DigiTech.
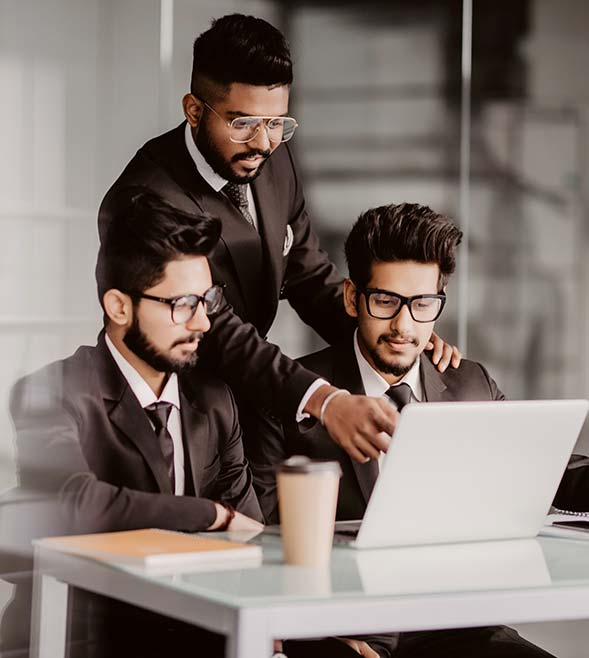
-
Direct Tax services
Our tax specialists offer a comprehensive blend of tax services, tax litigation, regulatory and compliance services, helping you navigate through complex business matters.
-
Indirect Tax Services
Get tax services by leading tax firm Grant Thornton India. Our indirect tax services include consulting, compliance and litigation services for corporate, international and transaction tax
-
Transfer pricing services
Our transfer pricing services experts provide a range of services from provision of APA services to handling large global assignments including Country by Country reporting.
-
US Tax
At Grant Thornton, we help individuals and dynamic companies deal with US tax laws, which are one of the most complicated tax legislations across the world.
-
Financial Services - Tax
Best financial advisory services, tailored for small and large businesses by the experts having comprehensive knowledge of domestic laws and access to multifaceted tools to provide a valuable results.
-
Financial Reporting consulting services
Our experts have significant hands-on experience in providing IFRS/US GAAP services, end-to-end solutions and support services to fulfil financial reporting requirements.
-
Fund accounting and financial reporting
International operations often lack standardisation and have varied local reporting formats and requirements. Our experts can offer proactive insights, practical guidance, and positive progress and help meet regulatory timeframes.
-
Compliance and Secretarial Services
Our experts can assist in overhauling the entire compliance machinery of the organisation through evaluation of the applicable statutory obligations, monitoring of adequate governance controls, reporting and providing ongoing support.
-
Global People Solutions
As businesses transcend borders, both domestic and global considerations need equal attention. Our interim CFO and financial controller support services help organisations meet the business vision.
-
Finance and accounting outsourcing
Our accounting experts assist organisations in managing their accounting and reporting. Our dedicated Integrated Knowledge and Capability Centre (IKCC), allows us to service both the domestic and global markets efficiently and cost-effectively
-
Compliance Management System
We have automation solutions for you that will allow meeting government requirements and remain diligent, which when failed, can lead to penalties and loss in revenue.
-
Global compliance and reporting solutions
At Grant Thornton Bharat, we meet the challenges of our clients and help them unlock their potential for growth. Our professionals offer solutions tailored to meet our clients’ global accounting and statutory reporting requirements. With first-hand experience of local reporting requirements in more than 145+ locations worldwide, we provide seamless and consistent international service delivery through a single point of contact.
-
Related-party transaction governance
Related-party transactions (RPTs) are common in business structures where organisations engage with their group entities, such as holding companies, subsidiaries, associate concerns, joint ventures, or key management personnel, for various operational or financial purposes.
-
Family Offices & Private Client Services
Grant Thornton Bharat Private Client Services offers tailored advisory for family-owned businesses, focusing on governance, compliance, tax, succession planning, and family office structuring to sustain wealth and preserve legacies across generations.
-
GTMitra: Tax & Regulatory Tool
GTMitra, a specialised tax and regulatory tool by Grant Thornton Bharat, supports multinational businesses in understanding laws and regulations for effective growth strategies.
-
Labour codes
Labour codes solutions help you transition through the new legislation. At Grant Thornton, we help businesses divide their approach to make sure a smooth transition.
-
Alerts
At Grant Thornton India, with the help of our tax alerts, we help to provide updates on how to minimise your tax exposure and risks.
-
Unlocking opportunities: India investment roadmap
The India Investment Roadmap resource is designed to navigate the complexities of Indian tax and regulatory laws, providing seamless guidance and a comprehensive set of solutions to ensure a smooth process for investors aiming to establish or expand their presence in India.
-
CFO Solutions
Our comprehensive suite of solutions for CFOs
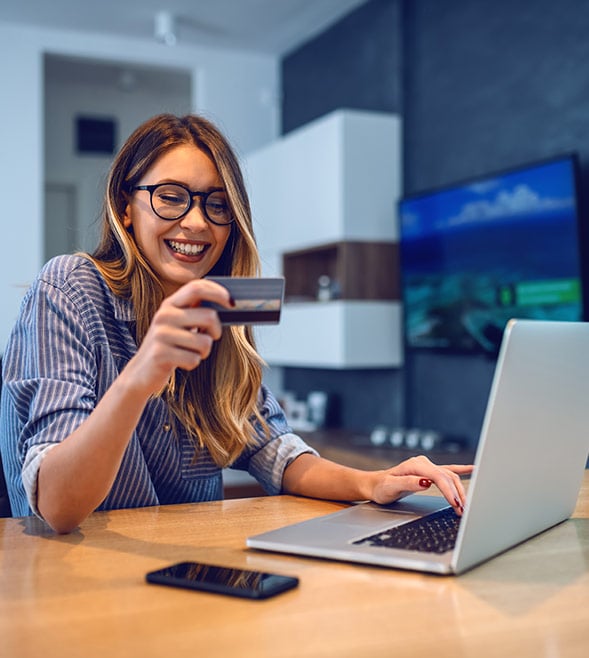
-
Cyber
In today’s time, businesses have gone through large transformation initiatives such as adoption of digital technologies, transition to cloud, use of advanced technologies et al.
-
Risk Optimisation
Our Governance, Risk and Operations (GRO) services encompass Internal Audit, Enterprise Risk Management, Internal Financial Controls, IT advisory, Standard Operating Procedures and other services.
-
Risk analytics
Grant Thornton Bharat’s CLEARR Insights is a state-of-the art data analytics platform that will help you in seamless data analysis and efficient decision-making.
-
Forensic & Investigation Services
The team of forensic advisory services experts consists of the best intelligence corporate experts, and fraud risk, computer forensic experts to deliver most effective solutions to dynamic Indian businesses.
-
Digital Forensics and Incident Response (DFIR)
Strengthen cyber resilience with proactive forensic audits
-
ESG consulting
Grant Thornton Bharat offers holistic ESG consulting solutions for sustainable business outcomes. With industry expertise and AI technology, we drive long-term value.
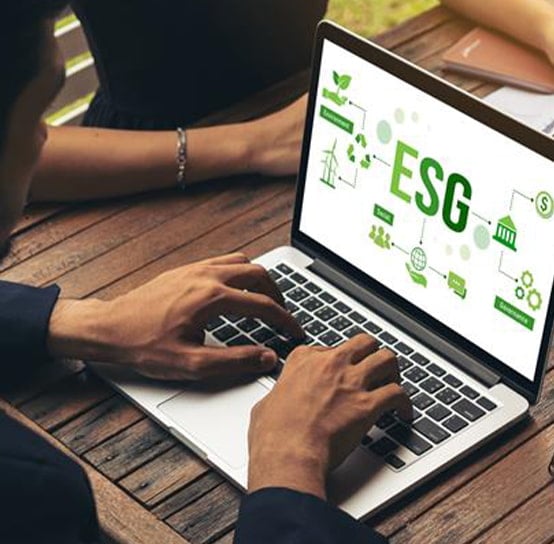
-
Transaction Tax Services
Our transaction tax experts understand your business, anticipate your needs and come up with robust tax solutions that help you achieve business objectives ensuring compliance and efficiency
-
Deal Advisory
Unlike other M&A advisory firm in India, we offer deal advisory services and work exclusively with controlled and well-designed strategies to help businesses grow, expand and create value.
-
Due Diligence
Grant Thornton’s financial due diligence services are aimed at corporate looking for mergers and acquisitions, private equity firms evaluating investments and businesses/promoters considering sale/divestment.
-
Valuations
As one of the leading valuation consultants in India, Grant Thornton specializes in all the aspects of the process like business valuation services, financial reporting, tax issues, etc.
-
Overseas Listing
Overseas listing presents a perfect platform for mid-sized Indian companies with global ambitions. Grant Thornton’s team of experts in listings, work closely with clients during all stages.
-
Debt & Special Situations Solutions
Grant Thornton Bharat offers specialist debt and special situations consulting services, including restructuring, insolvency, and asset tracing solutions.
-
Financial Reporting Advisory Services
Grant Thornton Bharat Financial Reporting Advisory Services offer end-to-end solutions for complex financial requirements, including GAAP conversions, IPO support, and hedge accounting advisory, ensuring accurate financial reporting and compliance.
-
Financial Statement Audit and Attestation Services
Grant Thornton Bharat offers customised financial statement audit and attestation services, ensuring impeccable quality and compliance with global standards. Our partner-led approach, technical expertise, and market credibility ensure effective solutions for your business needs.
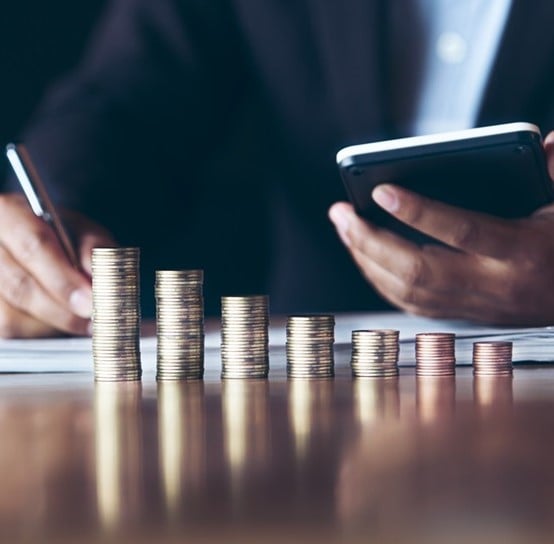
- Agriculture
- Asset management
- Automotive and EV
- Banking
- Education and ed-tech
- Energy & Renewables
- Engineering & industrial products
- Fintech
- FMCG & consumer goods
- Food processing
- Gaming
- Healthcare
- Urban infrastructure
- Insurance
- Media
- Medical devices
- Metals & Mining
- NBFC
- Pharma, bio tech & life sciences
- Real estate and REITs
- Retail & E-commerce
- Specialty chemicals
- Sports
- Technology
- Telecom
- Tourism & hospitality
-
Thought leadership Co-lending in India: Expanding credit access for MSMEsIn today’s rapidly evolving financial landscape, co-lending has emerged as a key enabler of credit expansion in India, facilitating partnerships between banks and non-banking financial companies (NBFCs) to extend credit more efficiently to underserved segments.
-
Article Why India’s financial inclusion journey needs to focus on equity and access to creditFinancial services have expanded over a decade, giving millions access to bank accounts and digital payments. But true empowerment needs to reach every corner of the country if growth is to be long-term and sustained
-
Thought Leadership Competitive and sustainable agriculture & food processing in KeralaThe economy of Kerala is primarily driven by the services sector, which contributes 66% to the Gross State Domestic Product (GSDP).
-
Article Economic Survey 2024-25: Deregulation, investment and innovation for a Viksit BharatIndia's economic growth remains for a steady trajectory with real GDP expected to grow at 6.4% in FY25 and in the range of 6.3%-6.8% in FY26, reflecting resilience despite global uncertainties.
-
Quarterly Aviation Insights
Explore the latest trends in aviation industry with Grant Thornton Bharat’s Quarterly Aviation Insights. Stay updated on industry growth, market shifts & key developments.
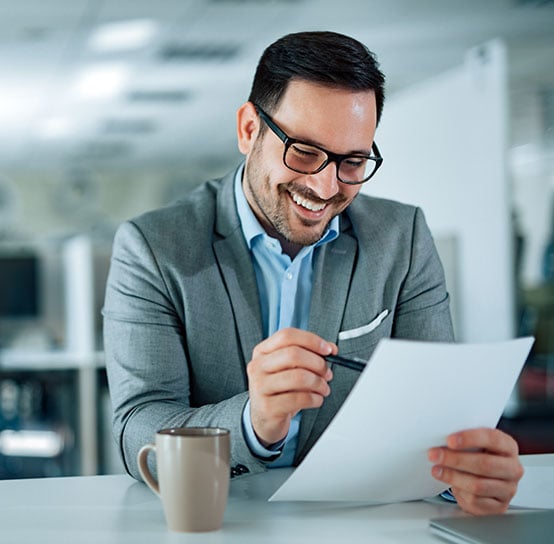
-
Freight Forward: Quarterly insights
Logistics sector in India is adapting to rising costs, global disruptions, and the growing urgency of sustainability.
-
India-UK
India-UK
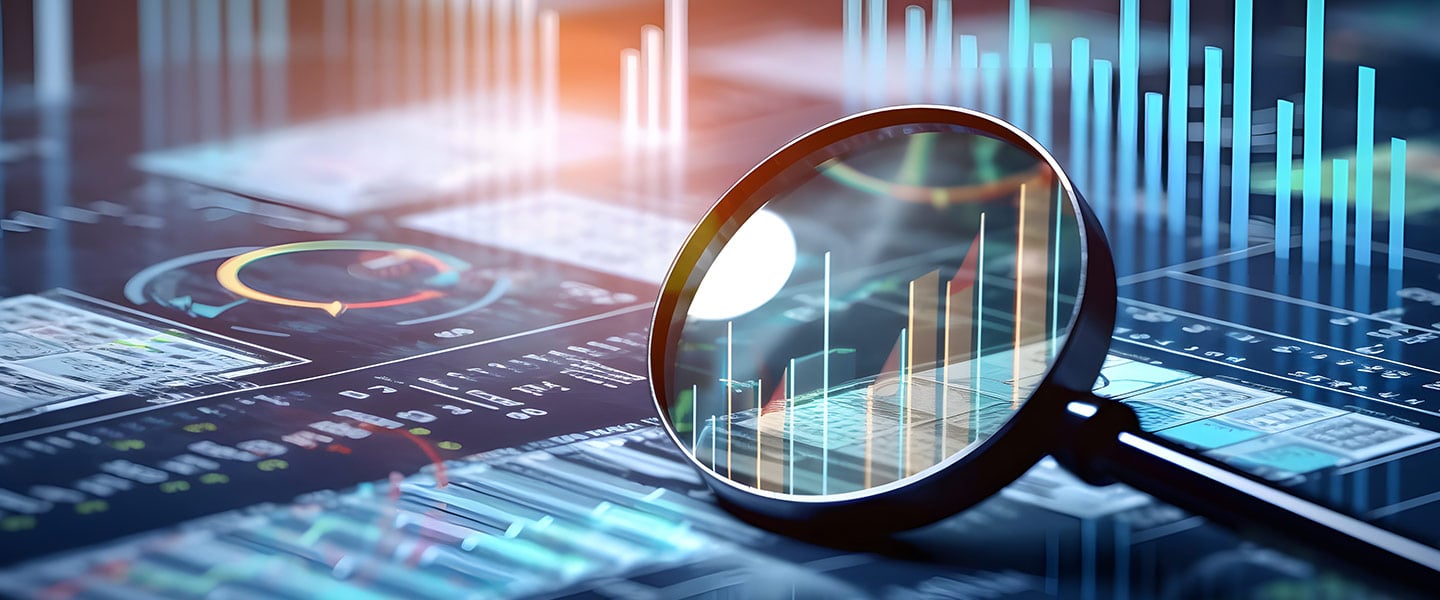
In the fast-paced world of modern business, the integration of a robust data strategy with advanced technologies is no longer a luxury, but a strategic necessity. Data strategy, the comprehensive plan outlining collection, storage, governance, and utilisation of data, forms the bedrock for informed decision-making. However, the recent emergence of Generative Artificial Intelligence (Generative AI or GenAI) adds a transformative layer to this landscape.
Defining Generative AI and its evolution beyond traditional AI
Generative AI is a significant development that moves beyond conventional artificial intelligence. Generative AI refers to AI systems that can generate new content or data. Unlike traditional AI, which relies on substantial historical data for analysis and decision-making, Generative AI goes a step further. It uses existing data to create new outputs, such as images, text or ideas. This evolution beyond traditional AI brings a level of innovation and creativity that can significantly impact data strategy.
AI and Machine Learning (ML) in data analysis:
With the integration of AI, data analysis has changed significantly. AI algorithms can now identify correlations, outliers and trends in large datasets, providing a deeper and more nuanced understanding of the information at hand. The speed and accuracy of data analysis are significantly enhanced by AI, empowering organisations to take informed decisions based on real-time insights.
Similarly, ML is used to forecast outcomes, recommend action, and automate routine decisions. This augmentation enables the leadership to focus on more strategic and complex issues, leveraging capabilities of AI and ML to enhance the overall decision-making framework.
Benefits of integrating Generative AI into data strategy:
The integration of Generative AI into data strategy brings forth several benefits, fundamentally changing the way organisations approach data analysis, decision-making, and strategic planning:
- Generative AI helps enhance data analysis by uncovering patterns and insights that may be challenging for traditional
- ML algorithms, fueled by Generative AI, identifies correlations, outliers, and trends in large This helps in better analysis and adds depth and complexity to insights.
- AI-generated insights, through the creation of new data, provides a more dynamic and forward-looking perspective. This, in turn, improves decision making by leadership, making them more accurate and
- Generative AI helps automate repetitive tasks and streamline data process workflows. Therefore, people become free for other strategic work and the efficiency and speed of data- related workflows
The table below highlights effects of AI integration into data processes. The difference AI integration brings to workflow is correlated to reducing redundant manpower and making processes efficient.
S. no | Data processes | Before AI integration | After AI integration |
1 | Data collection and acquisition | Focus on collecting structured data | AI automates collection, identifies patterns in unstructured data and optimises results. |
2 | Data storage and management | Creation of efficient databases and data warehouses | AI optimises storage, streamlines retrieval, and prioritises data based on usage. |
3 | Data analysis and interpretation | Relying on statistical methods and reporting tools | AL produces enhanced analytics with ML algorithms for identifying patterns and insights. |
4 | Decision-making processes | Relying on historical data and reports | AI augments decision- making with predictive analytics, recommendations, and automation of routine decisions. |
5 | Scalability and adaptability | Focus on handling increasing data volumes and adapting to changing business needs | AI automates tasks, adapts to evolving data sources, and ensures scalability and flexibility. |
6 | Data governance | Establishing policies for data quality, integrity, and security | This requires governance for machine learning models, including ethical guidelines and monitoring algorithmic bias. |
7 | Data security and compliance | Emphasis on security measures and compliance with data protection regulations | It involves securing AI models and complying with evolving AI regulations. |
While the integration of AI and ML into data strategy transforms the landscape of data-related processes, this transformation is not without its challenges.
Challenges and considerations:
Challenges of AI integration into data strategy can lead to ethical considerations, bias in algorithms, transparency in decision-making and the responsible use of AI. Furthermore, there is a debate of utilising AI and its benefits for success of the company and the welfare of the common public and concerns about being socially responsible, which is vital for overall stakeholder survival in the long term.
Emerging trends
As we look towards the future, we can speculate several trends related to integration of Generative AI. These are:
- Technological progress in Generative AI will continue to refine its capabilities. As models become more sophisticated and capable of understanding complex patterns, the ability to generate meaningful and innovative content is expected to reach new heights. This progress will contribute to a more nuanced and dynamic integration of Generative AI into data
- The evolving landscape of AI and particularly Generative AI will likely prompt regulatory changes and the establishment of industry standards. As the technology matures, regulatory bodies may also introduce guidelines and frameworks to ensure the responsible use of Generative
- The success stories of Generative AI in IT-led industries will likely drive increased adoption across sectors. From creative fields such as design and content creation to data-intensive domains such as healthcare and finance, organisations will recognise the potential of Generative AI to enhance their data strategies. The democratisation of Generative AI tools may also contribute to increased adoption, allowing organisations of all sizes to leverage its
Way forward
Summing it up, the integration of Generative AI into data strategy marks a significant leap forward in the evolution of business operations. It goes beyond the conventional boundaries of data analysis, decision-making, and automation. Generative AI actively contributes to the creative and innovative processes within organisations, opening new frontiers for strategic excellence. As organisations navigate this transformative journey, it is crucial to strike a balance between innovation and responsibility. Addressing ethical considerations, ensuring data security, and maintaining transparency in AI processes are essential steps in harnessing the full potential of Generative AI within data strategy.
For more information on our Data and analytics advisory services, check us out here.