-
Business Consulting
Our business consulting specialists offer a comprehensive blend of strategic advisory services. We assess the business, industry, operating model, synergy, skill sets and vision of the organisation and recommend the way forward
-
Digital Transformation Services
Grant Thornton’s digital transformation services help traditional businesses digitalise their business models with cloud technology, IoT consulting, app development and more DigiTech solutions.
-
Human Capital Consulting
Our Human Capital Consulting team harnesses technology and industry expertise to assist in constructing adaptable organisations with transparency, fostering productive and value-driven workforces, and inspiring employees to engage meaningfully in their tasks.
-
Production Linked Incentive Scheme
Production-linked Incentive Scheme by the Indian government is aimed at boosting manufacturing. Grant Thornton Bharat offers varied services across sectors to help businesses avail of this scheme.
-
Public Sector Advisory
Our Public Sector Advisory team has focused streams, aligned with the core priorities of the Government of India. We are responsible for providing innovative and customized technical and managerial solutions.
-
Tech Advisory
We have amalgamated Digital Transformation, IT Advisory & Information Management and Analytics into a new offering, DigiTech.
-
Direct Tax services
Our tax specialists offer a comprehensive blend of tax advisory, tax litigation, regulatory and compliance services, helping you navigate through complex business matters.
-
Indirect Tax Services
Get tax advisory service by leading tax firm Grant Thornton India. Our indirect tax services include advisory, compliance and litigation services for corporate, international and transaction tax
-
Transfer pricing services
Our transfer pricing services experts provide a range of services from provision of APA services to handling large global assignments including Country by Country reporting.
-
US Tax
At Grant Thornton, we help individuals and dynamic companies deal with US tax laws, which are one of the most complicated tax legislations across the world.
-
Financial Services - Tax
Best financial advisory services, tailored for small and large businesses by the experts having comprehensive knowledge of domestic laws and access to multifaceted tools to provide a valuable results.
-
Financial Reporting consulting services
Our experts have significant hands-on experience in providing IFRS/US GAAP services, end-to-end solutions and support services to fulfil financial reporting requirements.
-
Fund accounting and financial reporting
International operations often lack standardisation and have varied local reporting formats and requirements. Our experts can offer proactive insights, practical guidance, and positive progress and help meet regulatory timeframes.
-
Compliance and Secretarial Services
Our experts can assist in overhauling the entire compliance machinery of the organisation through evaluation of the applicable statutory obligations, monitoring of adequate governance controls, reporting and providing ongoing support.
-
Global People Solutions
As businesses transcend borders, both domestic and global considerations need equal attention. Our interim CFO and financial controller support services help organisations meet the business vision.
-
Finance and accounting outsourcing
Our accounting experts assist organisations in managing their accounting and reporting. Our dedicated Integrated Knowledge and Capability Centre (IKCC), allows us to service both the domestic and global markets efficiently and cost-effectively
-
Compliance Management System
We have automation solutions for you that will allow meeting government requirements and remain diligent, which when failed, can lead to penalties and loss in revenue.
-
IKCC: Grant Thornton's Shared Service Centre
The India Knowledge and Capability Centre (IKCC), aimed at delivering solutions by developing capabilities, has completed four years of its journey.
-
Global compliance and reporting solutions
At Grant Thornton Bharat, we meet the challenges of our clients and help them unlock their potential for growth. Our professionals offer solutions tailored to meet our clients’ global accounting and statutory reporting requirements. With first-hand experience of local reporting requirements in more than 145+ locations worldwide, we provide seamless and consistent international service delivery through a single point of contact.
-
Related Party Transactions Governance
Related Party Transactions Governance
-
Private Client Services
Private Client Services
-
Labour codes
Labour codes solutions help you transition through the new legislation. At Grant Thornton, we help businesses divide their approach to make sure a smooth transition.
-
Alerts
At Grant Thornton India, with the help of our tax alerts, we help to provide updates on how to minimise your tax exposure and risks.
-
Cyber
In today’s time, businesses have gone through large transformation initiatives such as adoption of digital technologies, transition to cloud, use of advanced technologies et al.
-
Governance, Risk & Operations
Our Governance, Risk and Operations (GRO) services encompass Internal Audit, Enterprise Risk Management, Internal Financial Controls, IT advisory, Standard Operating Procedures and other services.
-
Risk analytics
Grant Thornton Bharat’s CLEARR Insights is a state-of-the art data analytics platform that will help you in seamless data analysis and efficient decision-making.
-
Forensic & Investigation Services
The team of forensic advisory services experts consists of the best intelligence corporate experts, and fraud risk, computer forensic experts to deliver most effective solutions to dynamic Indian businesses.
-
ESG consulting
Grant Thornton Bharat offers holistic ESG consulting solutions for sustainable business outcomes. With industry expertise and AI technology, we drive long-term value.
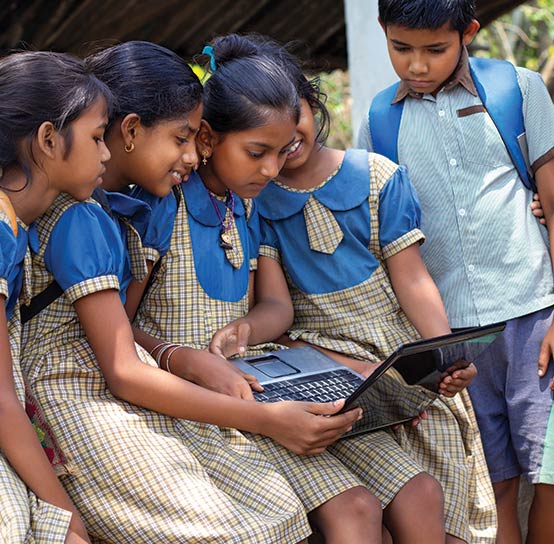
-
Transaction Tax Services
Our transaction tax experts understand your business, anticipate your needs and come up with robust tax solutions that help you achieve business objectives ensuring compliance and efficiency
-
Deal Advisory
Unlike other M&A advisory firm in India, we offer deal advisory services and work exclusively with controlled and well-designed strategies to help businesses grow, expand and create value.
-
Due Diligence
Grant Thornton’s financial due diligence services are aimed at corporate looking for mergers and acquisitions, private equity firms evaluating investments and businesses/promoters considering sale/divestment.
-
Valuations
As one of the leading valuation consultants in India, Grant Thornton specializes in all the aspects of the process like business valuation services, financial reporting, tax issues, etc.
-
Overseas Listing
Overseas listing presents a perfect platform for mid-sized Indian companies with global ambitions. Grant Thornton’s team of experts in listings, work closely with clients during all stages.
-
Debt & Special Situations Solutions
Grant Thornton Bharat offers specialist debt and special situations consulting services, including restructuring, insolvency, and asset tracing solutions.
-
Financial Reporting Advisory Services
Financial Reporting Advisory Services
-
Financial Statement Audit and Attestation Services
Financial Statement Audit and Attestation Services
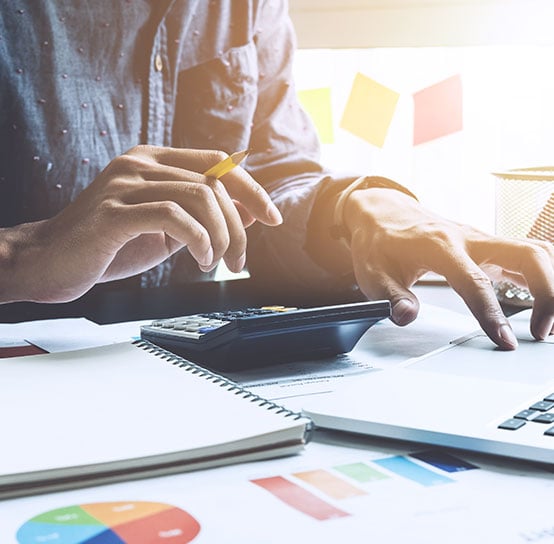
- Agriculture
- Asset management
- Automotive and EV
- Aviation
- Banking
- Education and ed-tech
- Energy & Renewables
- Engineering & industrial products
- FinTech
- FMCG & consumer goods
- Food processing
- Gaming
- Healthcare
- Urban infrastructure
- Insurance
- Media
- Medical devices
- Metals & Mining
- NBFC
- Pharma, bio tech & life sciences
- Real estate and REITs
- Retail & E-commerce
- Specialty chemicals
- Sports
- Technology
- Telecom
- Transportation & logistics
- Tourism & hospitality
-
India-UK
India-UK
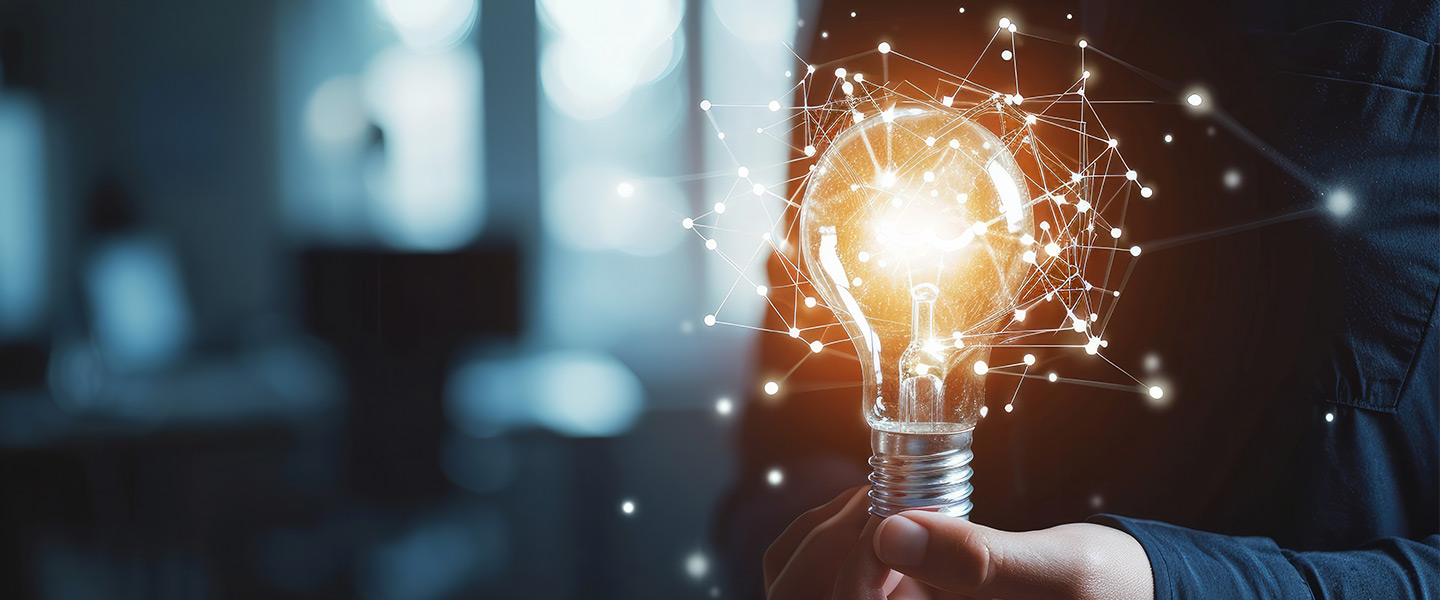
To establish a strong commitment to using data effectively, companies need a mix of people to handle the collection, organisation and analysis of data. The entire process, from gathering raw data to turning it into useful insights, is quite extensive and can get confusing - given its large volume. Companies inexperienced in handling data may unintentionally craft job listings that assign responsibilities to a job title in a way that goes against common expectations. On the flip side, job seekers may also find themselves in a predicament by pursuing opportunities that do not align with their skills or interests.
Thus, understanding the roles within data teams takes centre stage – primarily, machine learning (ML) engineers, data scientists, data analysts and data engineers. While these four are not the only data roles available, they are the most prominent ones and are the focus of most questions about distinguishing roles in data.
ML engineers:
The role of ML engineers revolves around designing, developing and deploying ML models to solve specific problems or enhance decision-making processes. They work closely with stakeholders to understand business problems and define clear objectives that can be addressed using ML tools. Following are some of their main functions:
- Model deployment: ML engineers design and create ML models tailored to solve specific problems or tasks. This involves selecting appropriate algorithms, optimising parameters and ensuring the model's accuracy. They serve as the architects of intelligent systems. They design and deploy models as per their firm’s requirements, ensuring that algorithms run seamlessly in real-world applications.
- Machine learning operations (ML Ops): ML Ops involves the deployment, monitoring and maintenance of ML models in production environments. ML engineers work on various aspects of ML Ops, including data infrastructure management, addressing scalability issues, version control systems to manage different versions of ML models and associated code, etc. In the context of ML Ops, these engineers streamline the entire lifecycle of ML activities. Additionally, they ensure that data pipelines are robust, enhancing the efficiency of model deployment and reducing the model’s time-to-market.
Data scientists:
Data scientists engage in exploratory data analysis (EDA) to understand the patterns, relationships and trends within the data. They also do feature engineering by creating new features or modifying existing ones to enhance the performance of ML models and data model evaluation. Following are some of their key responsibilities:
- Statistical and ML modelling: Data scientists blend statistical acumen with ML techniques. They create mathematical models that extract meaningful patterns from complex datasets. They also train these models using available data, after which they assess the model performance and validate their efficacy using various statistical metrics. They also interpret the results, identifying the most relevant features and understanding how the model makes predictions and if it aligns with resolving the problem statement or not.
- Inferences: Data scientists draw valuable inferences from data, providing actionable insights to guide business decisions. They also formulate hypotheses about the data and use statistical tests to evaluate the significance of observed patterns or differences. Data scientists then communicate their findings of complex mathematical analysis to non-technical/business stakeholders using clear and simple language. Visualisations, reports and presentations are often employed to convey complex results in a comprehensible manner to make the insights actionable.
- Experimentation: Experimentation is at the heart of data science. Data scientists work closely with ML engineers to experiment with various data models, innovative methodologies and new cutting-edge techniques, ensuring they align with the organisation's goals and solve the pain points. The iterative process involves constant feedback, trying out various options often derived from the deployment experience guided by ML engineers.
Data analysts:
Data analysts collect and clean data, conduct exploratory analyses and create visualisations to provide insights for decision-making. They use statistical methods, business intelligence tools and interpretation skills to present findings in reports and collaborate with stakeholders. The main difference between data engineers or ML engineers and data analysts is that the work of a data analyst starts after the data is collected, while data engineers or ML engineers often pick up incomplete and ‘messy’ sets of data to arrange, streamline, organise and work with. Following are some of the responsibilities of a data analyst:
- Business insights: Data analysts are storytellers who decipher the language of data for business stakeholders. Collaborating with data scientists, they translate complex models and analyses into actionable insights, facilitating informed decision-making. They also validate the accuracy and reliability of data. They also play a pivotal role in establishing data quality standards and implementing processes to maintain data integrity.
- Metrics and reporting: Data analysts focus on defining and monitoring key performance indicators. Their collaboration with data engineers ensures that the metrics tracked are derived from accurate and up-to-date data sources, underlining the importance of a seamless data pipeline and data traceability.
- Data visualisation and storytelling: The art of data-based storytelling is mastered by data analysts. They collaborate with ML engineers and data scientists to create visual narratives that make complex insights accessible to a broader audience, fostering a data-driven culture within organisations.
Data engineers:
Traditionally, companies hire data engineers when they want to work with data at the foundational level. Data engineers play a crucial role in collecting, organising and maintaining data, making them essential to the principal steps of handling organisational as well as external data. Their role includes:
- Design data pipelines: Data engineers are the architects of data infrastructure. They collaborate with ML engineers to design robust pipelines that feed data models with high-quality data, ensuring the success of models and their deployment.
- Optimise databases and metadata: Databases form the backbone of data models, storage and retrieval. Data engineers ensure that the databases are optimised for storage, metadata is efficiently designed, and retrieval of data needed for model training and inferences is quick and less resource-intensive.
- Develop data tools: Data engineers develop and maintain the tools necessary for seamless data extraction, wrangling and processing. Collaboration with data analysts ensures that the tools are user-friendly, enabling analysts to extract insights efficiently.
ML engineers, data scientists, data analysts and data engineers, each with their unique responsibilities, come together to create a holistic data ecosystem. Their collaboration in the process not only ensures the smooth functioning of individual roles but also fosters innovation, making the data landscape a seamless, well-oiled machine. As organisations increasingly acknowledge the pivotal role of data, the symbiotic relationships among these roles will inevitably progress, expanding on what can be accomplished through the strategic utilisation of information. As the data ecosystem evolves further and there are more technological innovations (including artificial intelligence), the lines between these roles may get blurred but the constituent skills surely will be skills worth investing in in the future.
For more information on our Data and analytics advisory services, check us out here.